Using big data and AI to inform airport management strategies
- Like
- Digg
- Del
- Tumblr
- VKontakte
- Buffer
- Love This
- Odnoklassniki
- Meneame
- Blogger
- Amazon
- Yahoo Mail
- Gmail
- AOL
- Newsvine
- HackerNews
- Evernote
- MySpace
- Mail.ru
- Viadeo
- Line
- Comments
- Yummly
- SMS
- Viber
- Telegram
- Subscribe
- Skype
- Facebook Messenger
- Kakao
- LiveJournal
- Yammer
- Edgar
- Fintel
- Mix
- Instapaper
- Copy Link
Posted: 21 July 2021 | Florian Bertosio, Julien Nizzardo, Marc Houalla | No comments yet
Marc Houalla, Deputy Executive Director of Groupe ADP and Managing Director of Paris‑Charles De Gaulle Airport, argues that big data and artificial intelligence is becoming increasingly essential for airport management and takes an in-depth look at how it benefitted operations within his own airport.
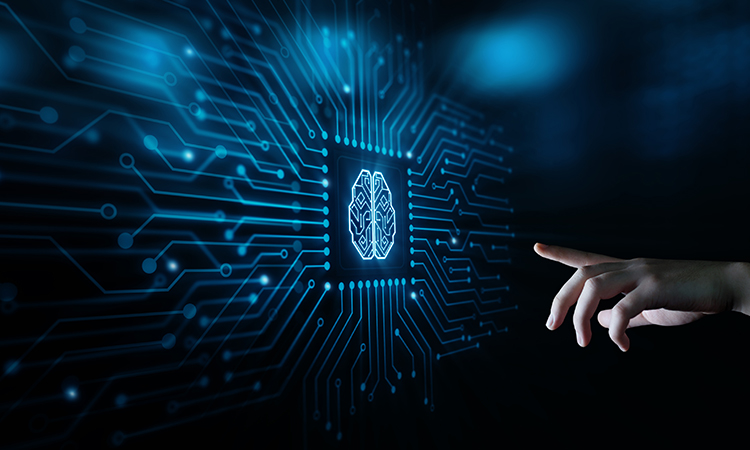
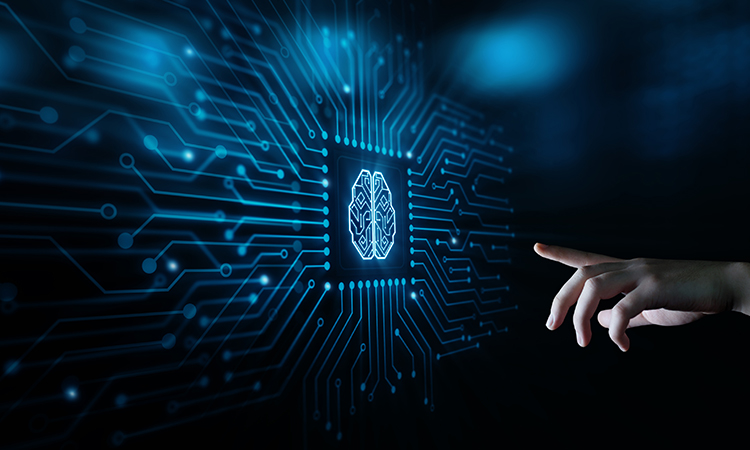
Paris-Charles de Gaulle Airport (CDG) is now using big data and artificial intelligence (AI) technologies to accurately forecast passenger flow at security control lines and passengers with reduced mobility (PRM) booths in its terminals, weeks and days in advance. With these advanced technologies, Paris-CDG is also able to diffuse bottlenecks caused by the heavy traffic flow of passenger cars at the airport throughout the day. This initiative has proven to be successful in minimising the trade-off between passenger experience and the cost of managing airport processes.
Past problems
One of the main performance indicators of passenger service quality is the waiting time during various airport processes, such as flight check-in, immigration or security controls, etc. Allocating extra human resources across the board is one sure way to reduce bottlenecks and, thus, improve the passenger experience. This, however, results in additional costs.
Before using big data technologies, the methods for predicting passengers flow at security control lines and PRM booths were empirical. Information on flight carriage rates was sent to multiple operational units that are responsible for passenger flow forecast, as well as human resources at the security control lines and PRM booths. These operational units would then apply empirical modifications to flight carriage rates to come up with new forecasted passenger flows. All modifications (based on airline connections, the geographic origin or destination of passengers, or the date and time of flights) were performed in an Excel tool. This Excel tool was difficult to maintain and had limited capability in processing large data.
Paris-CDG also experienced road accessibility problems during the day with peak flows. Traffic congestion on the road leading to the airport often delayed access to the car parks, resulting in stress and dissatisfaction. There was no accurate model for predicting road congestion, nor any effective evaluation practices for alleviating congestion.
Big data analysis
In order to design a robust forecasting model, it is important to determine the so-called ‘explanatory’ and ‘explained’ variables that will allow algorithms to make the most accurate forecasts of future passenger or vehicle flows.
In the case of the passenger flows analysis at security checks or PRM booths, the explained variables are the number of passengers arriving by intervals of 10 minutes. The associated explanatory variables consist of the flight numbers of passengers, the dates of flights (taking into consideration specific dates, such as public holidays, weekends, IATA seasons) and flight time, etc.
Studies on road flows pinpoint the correlation between the vehicle travel time (from the entrances of the airport to the car parks) with the following factors: passenger flows in the next two to three hours; the number of flights within two to three hours; vehicle traffic flows at the car parks in the previous hours; and the days of the week. For example, big data analysis demonstrates that the weekend drivers generate more congestion on the airport roads than weekday drivers.
The analysis and development of these models were conducted with the following algorithms: Random Forest, LSTM (Long Short-Term Memory) neural networks and the classic error measurement function, MSE (Mean Square Error).
Outcome
Through the big data model, Paris-CDG has improved its passenger flow forecasts at the security check points, as shown in Figures 1 and 2.
The daily flow at a security line at Paris-CDG (Figure 1) shows that the predicted passenger flows generated by the big data model match closer to the real passenger flows than the previous Excel model.
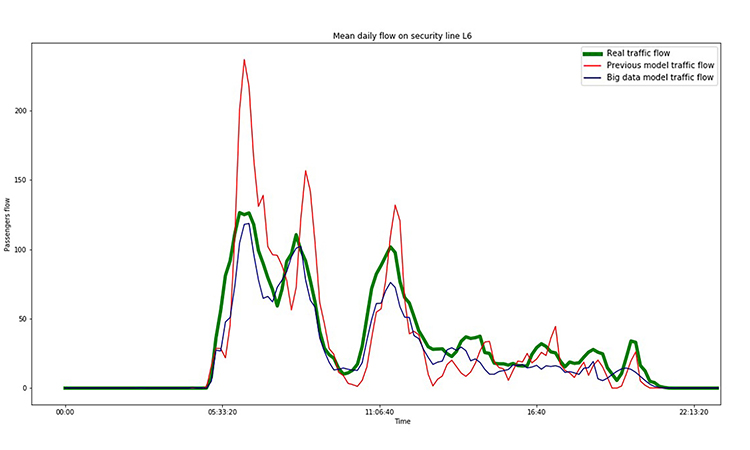
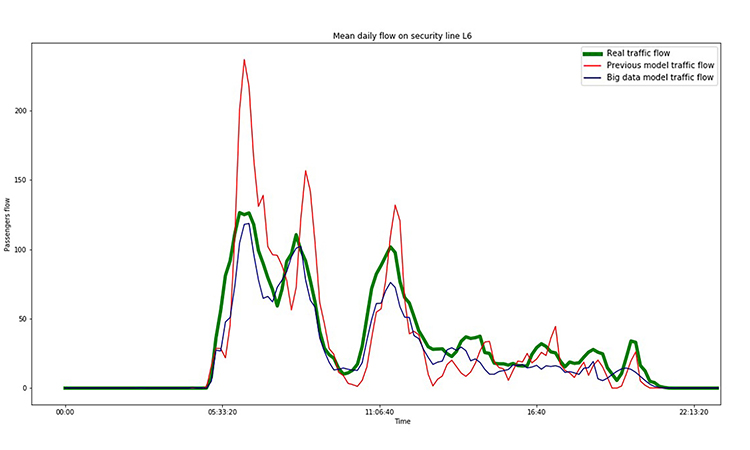
Figure 1: Comparison of passenger flows curves (real, previous model, big data model).
When comparing the mean error (error being the difference between the model passenger flow and the real one) of the big data and Excel models versus actual passenger flow in March 2019 (Figure 2), it confirms that the big data model shows less errors than the previous Excel model. When applied to all security lines at Paris-CDG, the big data model consistently exhibits superior forecasting capabilities for passenger flows.
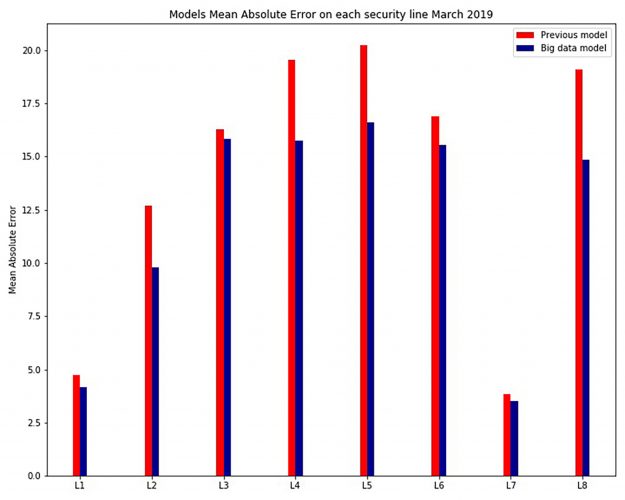
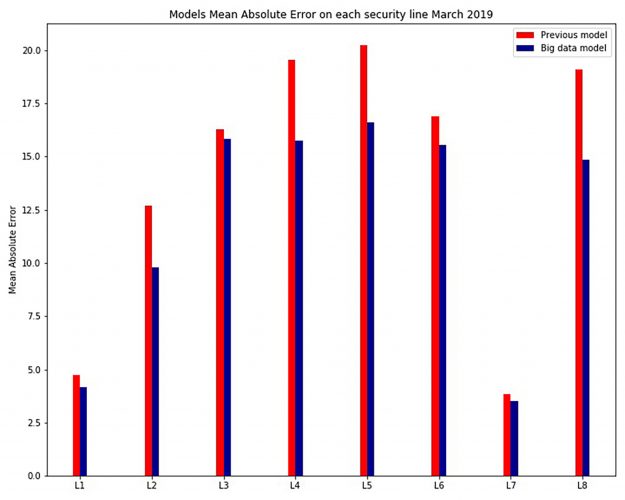
Figure 2: Mean absolute error (real passenger flows versus model passenger flows on security lines L1-L8).
This improvement in the ‘10-minute steps’ passenger flows forecast on security checks has enabled optimal allocation of staff at the control points. This has resulted in a reduction of millions of euros in security costs, as well as passenger waiting time.
The car traffic model, drawn from the use of big data technologies, has also made it possible to evaluate the effectiveness of traffic measures that are aimed at reducing car traffic jams. For example, it improves the efficiency of passenger drop-off zone barrier gates during specific periods and of many other practices, allowing operational staff to focus on the implementation of the most effective processes. In this case, the use of big data technologies halved the travel time from the airport entrance to the car parks.
The application of the big data algorithm for forecasting PRM flows was slightly disappointing, as it did not significantly improve the existing models. However, the Groupe ADP big data team is currently improving the PRM model by modifying the explanatory variables, the size of aggregated data sets and the use of other algorithms, such as neural networks.
Lessons learned
Paris-CDG’s first use of big data technology to tackle operational problems highlights three main lessons.
Since the application of big data has proven to be successful, operational staff are now raising additional topics that are in need of analytical techniques”
Firstly, involvement of the on-the-job staff is a prerequisite, not only for the launch of any big data initiative – in particular for the definition of the application cases – but also throughout experiments and the interpretation of the models results. On-the-job staff are best poised to validate or challenge the results obtained through big data techniques. In fact, they are the ones who originated the request of big data analysis, since the data flow that they had to manage exceeded their tools’ capacities. Since the application of big data has proven to be successful, operational staff are now raising additional topics that are in need of analytical techniques.
The second lesson shows that, when the volume of data is substantial, it is essential to ensure the integrity, relevance and accuracy of data and to systematically collect and classify the granularity of data prior to producing final analyses.
Thirdly, the use of big data technologies requires cross-functional skills. Big data use cases, presented in this document, precisely highlight this point. Data architects are needed to build an architecture capable of ingesting data and providing the computing power required for the application of the algorithms. Once the data is made available, its consistency should be ensured through the skills of data engineers. Data analysts/data scientists are essential, as they possess skills in data analysis, from analytical, algorithmic, statistical and mathematical points of view. Within Groupe ADP, these two profiles are currently represented in various business operating entities and within the Information Systems Department. However, if those skills are not available within an airport’s resources, one could engage external services or partner with specialised data science engineering universities.
Conclusions
Conventional data analysis and mathematical models for airport processes have reached their limits beyond a certain volume of data. In that case, the use of big data technologies could be of great help and even, sometimes, essential to analyse airport processes. The three big data cases, presented in this article, target the fluidity of the passenger journey, which is one of the airport’s operational activities’ core businesses. In addition, these technologies and techniques can also cater for the needs of other airport activities, such as predictive maintenance, luggage management and retail, etc.
In essence, big data and artificial intelligence could present a real competitive advantage for airports, especially in crisis situations, during which they can accelerate recovery time, leveraging agility in activities and processes, while maintaining optimal passenger experience. Data science demonstrates its cross-functional usefulness for airport management and its increasing importance in any company’s strategy.
Issue
Related topics
Airside operations, Big data, New technologies, Passenger experience and seamless travel, Passenger volumes, Passengers with reduced mobility (PRMs), Security, Terminal operations