The value of data analytics for airports: Use cases and novel methods
- Like
- Digg
- Del
- Tumblr
- VKontakte
- Buffer
- Love This
- Odnoklassniki
- Meneame
- Blogger
- Amazon
- Yahoo Mail
- Gmail
- AOL
- Newsvine
- HackerNews
- Evernote
- MySpace
- Mail.ru
- Viadeo
- Line
- Comments
- Yummly
- SMS
- Viber
- Telegram
- Subscribe
- Skype
- Facebook Messenger
- Kakao
- LiveJournal
- Yammer
- Edgar
- Fintel
- Mix
- Instapaper
- Copy Link
Posted: 7 April 2021 | Nikos Papagiannopoulos | 1 comment
Nikos Papagiannopoulos, Senior Project Manager at Athens International Airport, discusses the role of airport data analytics as an enabler to unleash key information, how this is used across the aviation industry and how it has the potential to solve significant airport challenges.
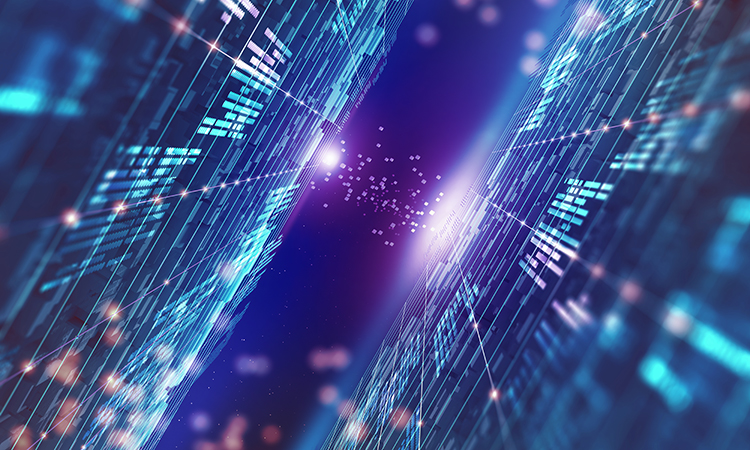
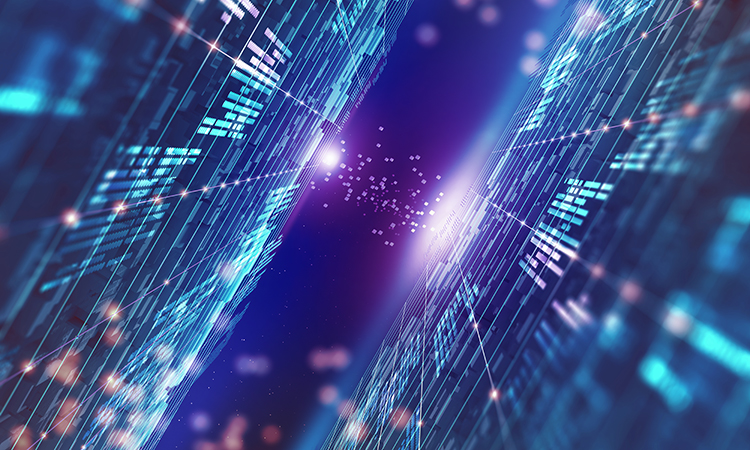
Many airports have defined and implemented highly efficient and effective processes to perform aircraft, passenger, baggage and cargo operations. Having reached high standards of airport operations, the room for improvement diminishes, while the effort to identify, define and test novel processes is increasing exponentially. Traditional methods that rely on empirical knowledge, observation and scenario testing are not expected to produce, anymore, the operational benefits that they have managed to attain in the past. Furthermore, there is a need for evidence-based substantiation of empirical airport operational knowledge in an effort to quantify and measure the operational benefits that have been attained and explore whether there is any opportunity to further augment those processes.
Airport data analytics are considered as the enabler to unleash key information and knowledge that will help to address many of the key areas where airports face challenges. These areas extend to almost every facet of airport processes, from purely operational ones – such as airport resource usage optimisation, maintenance and airport capacity – to non–aeronautical revenue intensification and passenger experience maximisation.
The unprecedented volume, diversity and richness of aviation data that can be acquired, generated, stored and managed provides unique capabilities for aviation-related industries (airlines, airports, passengers, service providers, manufacturers, local authorities, etc.) and pertains value that remains to be unlocked. In fact, big data from airlines, airports, aircrafts and extra-aviation service providers, combined with open linked data (e.g. for weather, environment, population, etc.), have the credentials to reassess the mentality of the aviation ecosystem by predicting early critical failures and maintenance needs, optimising flight paths, rescheduling routes in real-time, improving operational efficiency, serving a seamless ground/air passenger experience, safeguarding the environment and monitoring safety and risk threats (like epidemics and terrorist attacks).
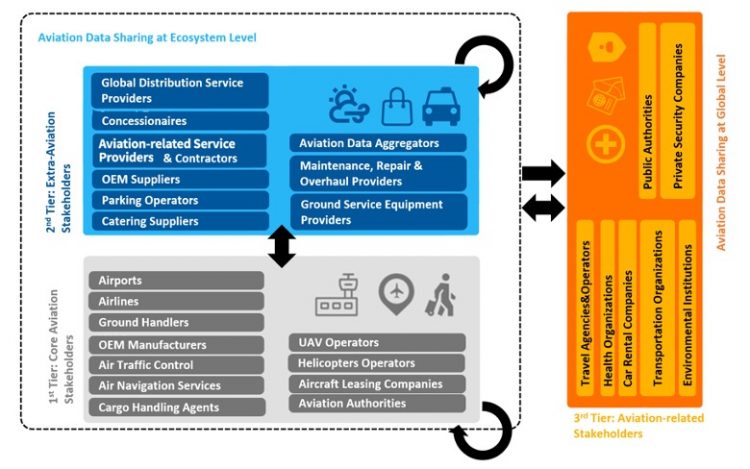
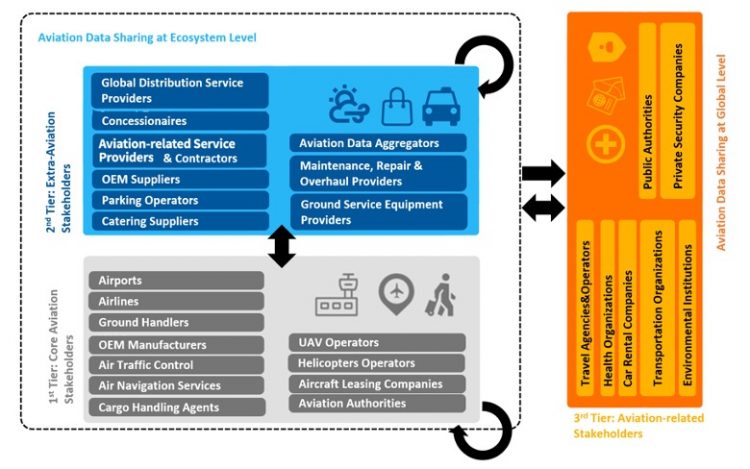
Figure 1: Aviation value chain stakeholders.
Today, despite the large investments into mining data and exploring analytics in the aviation industry, there is little data diffusion and sharing between the different stakeholders of the aviation-related sectors. Typically, organisations (e.g. airlines, airports, extra-aviation service providers, local authorities, health agencies, etc.) only have fragmented data at their disposal and still operate in silos, without effectively managing to link their data assets and gain better insights.
To this end, Athens International Airport (ATH) has joined the ICARUS Horizon 2020 research and innovation project, in order to help to develop and validate those methods that will address the strong market need for trusted and fair data sharing; affordable and cost-efficient data linking; and insightful and understandable data analytics in the aviation data value chain, in alignment with the recommendations of a number of policy and industry-specific initiatives.
Data analytics and the aviation landscape
The purpose of data analytics in aviation is to examine the vast amount of data generated daily and provide useful information to airlines, airports and other aviation stakeholders so that they can improve their operational planning and execution, as well as any related products and services.
Nowadays, aviation data derives from diverse sources and usually lacks the standardisation, uniformity and fault controls required for reliable integration in a common analytics platform. A mere examination of the relative literature shows an evolving domain that takes data collection and mining very seriously but faces a real obstacle when it attempts to scale up and combine data sources. In order to cope with this challenge, many participants in the aviation industry have implemented their own isolated solutions, which may improve particular processes but fail to capture the whole picture.
Today, with the use of big data analytics and technologies, the accumulation and processing of massive datasets has become easier, while, at the same time, the increasing application of machine learning techniques has led to advanced predictive analytics, extracting trends and insights from heterogeneous data sources able to address modern operational needs and requirements.
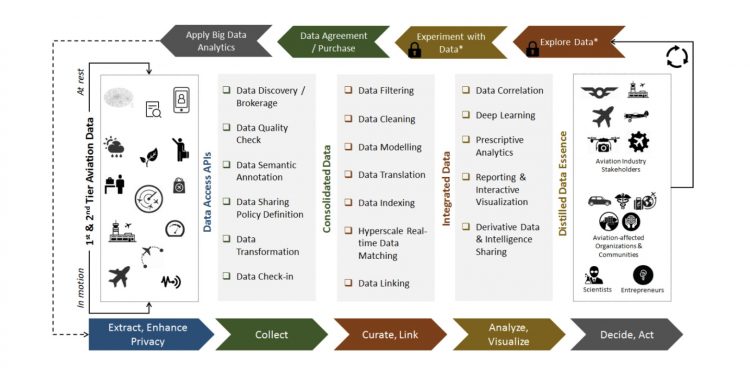
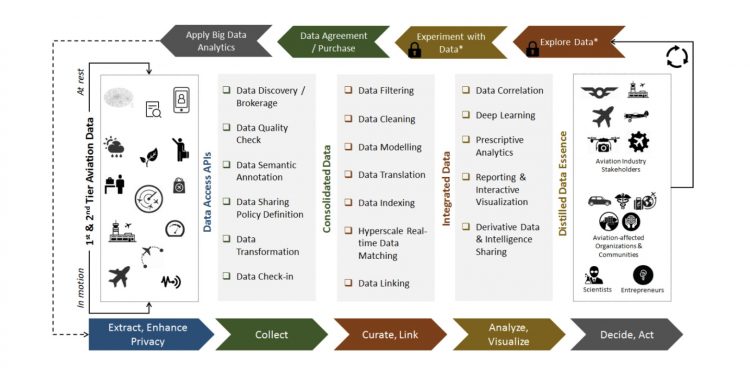
Figure 2: Icarus data analytics and sharing platform.
Athens International Airport use cases
Athens Airport has defined its use cases with the outlook of optimising airport capacity planning under real-time operations in order to understand the current airfield performance and to properly perform an analysis that will eventually lead to the improved seasonal planning of operational resources. Historical data for the runway, aprons, gates and aircraft stands (from airport stakeholders and certified aviation organisation sources) were leveraged together with aviation-related open data sources.
The capacity of the airport infrastructure (stands, gates vs planned aircraft arrivals) is generally adequate to meet the demand of airport users from a seasonal planning perspective; but, when it comes to pre-tactical and tactical planning during a busy day, and especially at peak hours, any kind of flight schedule disruption (i.e. related to late arrivals or weather) might have a significant impact on on-time performance.
The objective of the airport demonstrator is to improve the overall airport resources planning in an evidence-based, data-driven manner. With the help of the data analytics platform, the airport addresses the optimum airport capacity planning to ensure the most efficient use of the airport infrastructure, aiming at a sustained increase in throughput performance, given the available capacity, under all modes of routine/non-routine operations and weather conditions.
ATH plans to run various descriptive and predictive analytics to address common challenges, such as Flight Delay Prediction model definition. In particular, data analytics will allow the airport to discover hidden patterns and implicit relations between operations and scheduling, and learn from past experience in order to:
- Analyse and suggest enhancements on the planning of airport resources (stands, gates and check-in counters), taking into account flight schedules (submitted by aircraft operators) by early identifying the airport’s high and low traffic periods and through traffic forecasting at an hourly level
- Achieve optimum utilisation of ground services equipment in collaboration with ground handlers through more accurate and timely predictions of future delays based on the airline, the route and the type of the aircraft, taking also into account the seasonality (day and time, as well) and the weather
- Optimise the airport operation by effectively optimising the seasonal, as well as pre-tactical/tactical, planning of operational resources at a given day.
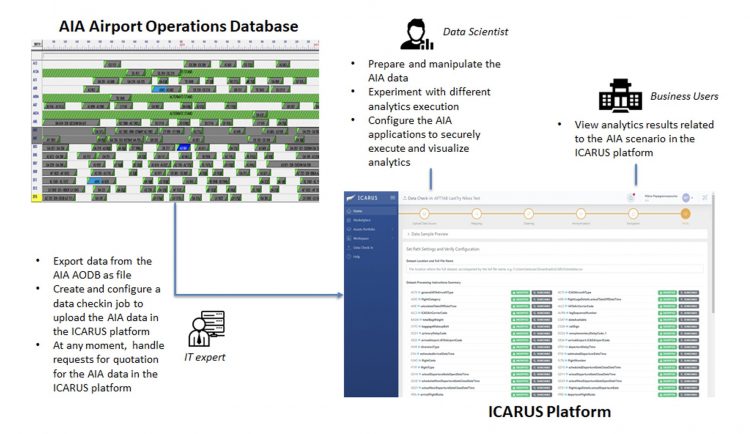
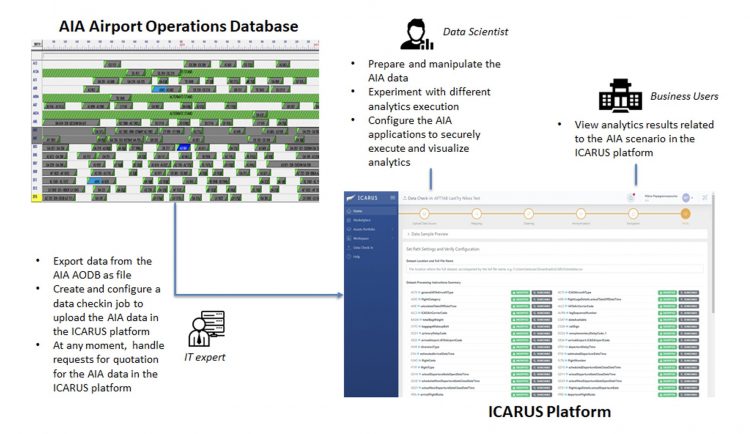
Figure 3: ATH’s AODB and ICARUS platform interaction.
In order to effectively use the data analytics platform, the airport extracts the necessary data from its Airport Operations Database (AODB) and its IT users upload them in the data analytics platform, performing all necessary configurations. The airport data is available in the platform, explored and analysed by a data scientist in order for the business/operational users that eventually access the ICARUS platform to view the results of the analysis that affect their operations.
The need for big data in aviation and the impact of COVID-19
In recent months, the shock and impact of the COVID-19 pandemic has hit all of the aviation sector and related services. While it’s hard to analyse the true longer-term impact, many analysts have already underlined how organisation and efficiency are two of the key factors for the recovery of the sector.
Challenges such as airport congestion, weak-business continuity plans, the need for social distancing and having as many ‘touchless’ interactions as possible suggest that there could be benefits from leveraging IT and big data – and related services.
At the same time, COVID-19 is, unfortunately, still an ongoing emergency all over the world, carrying with it a strong degree of uncertainty and difficulty to assess longer-term impact on most sectors, including aviation.
Key considerations for data analytics in aviation
The previous sections provide some preliminary insights regarding the role of data analytics in various aviation-related use cases and highlight the potential benefits across the complete value chain. The decision to adopt such processes also raises certain considerations:
Data availability
As revealed by the term data analytics, data constitutes the most significant part of the process. There is an underlying need to feed any data analytics process with appropriate data. The word appropriate is hard to define in this context, as it is not limited to the data content. Data structures, data veracity, data quality and, most of all, data availability and completeness need to be examined prior to any data analytics application. The data also significantly influences the selection of the most suitable algorithm and the pre-processing is, in many cases, the most demanding part of the whole process.
Type of problem
It should be stressed that not all problems can or should be expressed as data analytics problems. Regarding machine learning, in particular, whose advantages were individually examined and discussed as it is a very powerful data analysis method, it should be stressed that approaches that fall under the operations research category may be more suitable for certain problems.
Having concluded that a given problem can benefit from a data analytics solution, the next important consideration is how to select the most appropriate one, or how to combine some models in a hybrid solution. However, identifying the right option is a multifaceted problem and no predefined answers can be provided.
Stakeholder perspective
As shown in Figure 1, the aviation value chain is complex, and use cases are highly interconnected and assumptions and simplifications are often required in order to examine a certain problem in isolation. Even then, each problem can be seen from diverse perspectives depending on the stakeholder who acts as the decision-maker. As in most business-to-business transactions, the objective of each involved party may differ; in this case, resulting in a different formalisation and modelling of the same underlying processes. This fact may significantly impact not only the specified objective of the data analytics process and possible constraints, but also the selection of the most appropriate algorithm.
Technical issues
Computational needs, especially in a big data context, should be taken into consideration, and appropriate infrastructures should be foreseen when developing a data analytics solution. Furthermore, other aspects – such as the integration of data sources and extensibility of the adopted solution – can also pose significant technical challenges along the way.
Data analytics mentality
The data analytics concept in an industry perspective should not be treated as a must-have because it is a trend hype, especially in a complex environment like the one formed by the interacting aviation stakeholders. This would create unrealistic expectations and would push involved parties to develop rushed solutions, hindering the adoption of a truly beneficial data analytics strategy. Biases hidden in the data, datasets that fail to represent properly the underlying situation and overlooked correlations are almost certain to appear in the first steps of a data analytics model development. A successful data analytics approach requires time and the will to experiment, evaluate and refine the developed models. Data analytics should, therefore, be considered part of the core business processes.
Footnote
As the Icarus project is reaching its final phase, you are invited to follow the progress in the project at the relevant website. The Icarus platform is about to be released for experimental use to competent users; therefore, if you are interested in obtaining early access to the ICARUS beta platform, please contact this email address.
The project is co-funded by the European Commission Horizon 2020 – Grant #780792 , ICT-14-2017: Big Data PPP: cross -sectorial and cross lingual data integration and experimentation.
Nikos Papagiannopoulos is a Senior Project Management professional at Athens International Airport, with 20+ years of experience in mission critical airport IT projects. He is also responsible for IT Research & Innovation projects in the context of FP7 & EU Horizon 2020 programmes. Papagiannopoulos is an expert in the areas of data management and analytics, cyber-security, the Internet of Things (IoT), 5G mobile networks, IT cloud and digital transformation projects. He is also PMP, CISA, CISSP, ISO 27001 LA and ITIL certified.
Related topics
Airside operations, Big data, COVID-19, New technologies, Terminal operations
Great overview of how big data shared by various industry partners can contribute to efficiency and capacity. By so doing there is significant potential not only for cost savings but improved efficiency is likely to also improve safety and reduce environmental impact. EASA’s Data for Safety (D4S) project could be a next step to identify further use cases.