Data analytics are driving a bright future
- Like
- Digg
- Del
- Tumblr
- VKontakte
- Buffer
- Love This
- Odnoklassniki
- Meneame
- Blogger
- Amazon
- Yahoo Mail
- Gmail
- AOL
- Newsvine
- HackerNews
- Evernote
- MySpace
- Mail.ru
- Viadeo
- Line
- Comments
- Yummly
- SMS
- Viber
- Telegram
- Subscribe
- Skype
- Facebook Messenger
- Kakao
- LiveJournal
- Yammer
- Edgar
- Fintel
- Mix
- Instapaper
- Copy Link
Posted: 28 May 2020 | Floris Herrema - EUROCONTROL | No comments yet
For International Airport Review, Data Scientists at EUROCONTROL analysed recent studies, such as EUROCONTROL’s Challenges of Growth, to offer a glimpse into how data can be used in the future of ATC/ATM.
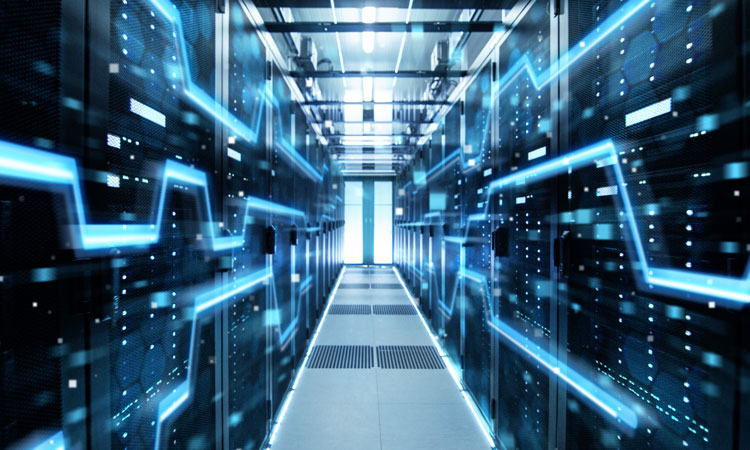
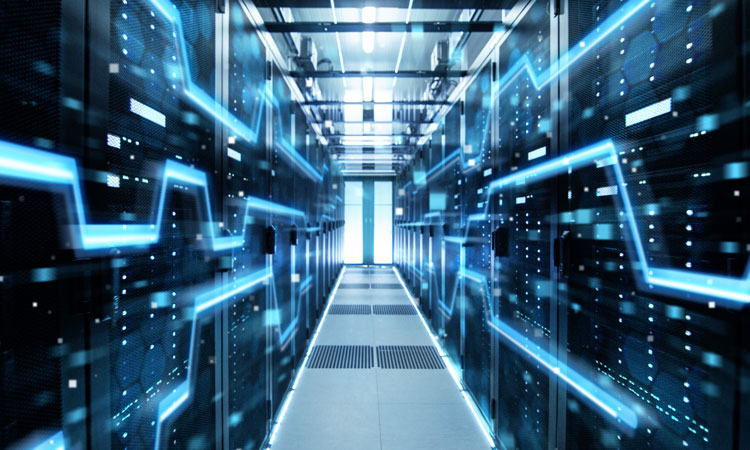
A variety of studies have recently forecast continued long-term growth in traffic demand, with resulting increases in delays and constrained access to airports. The cost to the European economy and impact on the travelling public is forecast to be billions of euros.
Operational improvements under development in the European ATM Research Programme, SESAR, will bring some respite. However, researchers are turning to the power of big data, artificial intelligence and the sub-domain machine learning to boost efficiency and operational performance. Data-related improvements on runway throughput, for example, can result in five to 10 per cent additional capacity at peak traffic times for a relatively affordable investment.
The application of machine learning (ML) is one area being vigorously researched. ML provides a system with the ability to learn by looking for patterns in data that can result in accurate predictions.
Data-driven predictions of future events will support smart strategies to manage passenger and aircraft flows, such as passengers moving through airport terminals, boarding aircraft and air traffic arrival sequence predictability – they will help pilots and air traffic controllers to focus on priority tasks. This is very dependent on achieving optimal human/machine teaming, and being able to easily explain the ML application.
However, ATM remains a safety-critical industry, and data analytics applications require rigorous research to ensure that they are capable of meeting the high safety and security requirements of aviation. Verifying and validating ML-related operational improvements in the live ATC environment remains a significant challenge for regulators. Nevertheless, we must not forget performance benefits can be had from non-safety critical applications.
Maturing data analytics in EUROCONTROL SESAR Research: Runway performance
Recognising the increasing opportunity of data in predictive applications, EUROCONTROL – together with stakeholders in areas such as airspace, network management and runway management techniques – is actively investigating the use of data analytics to identify performance improvements and benefits.
Some early applications investigated the predictability of aircraft turnaround at the gate and taxi-out time (TXOT) in support of performance prediction tools to be used in an Airport Operations Centre (APOC). The expectation is that, with access to accurate data, decision-making is improved and operational staff become more proactive.
One application tested produced TXOT predictions using data from Paris-Charles de Gaulle, which improved the prediction robustness compared to traditional statistical analyses. Furthermore, TXOT predictions were quickly computed in a few minutes.
Another application, developed in the EU SafeClouds project, predicted the runway exit to be used after landing during high intensity runway operations in order to help tower controllers judge separation minima between leader and follower aircraft, thus optimising runway occupancy time and reducing potential go-around or missed approaches.
In the runway exit application, a prediction is produced at 2NM from the runway threshold and presented to the controller through a runway tactical support tool. This tool advises the controller of the predicted arrival runway occupancy time (AROT) and runway exit for each aircraft, helping the controller to anticipate any separation reduction for the following arriving aircraft.
The ML runway exit support tool was based on Vienna airport and tested in a SESAR Real Time Simulation undertaken by EUROCONTROL. Vienna controller Philipp Wächter, who took part in the simulation, said that the “controller support tool for AROT and runway exit prediction was considered operationally feasible and acceptable”.
Whilst this work will be continued to pre-implementation level in SESAR, a real-time prototype was later developed and tested with data from Orly Airport, with critical wind data provided by a ‘Leosphere’ Lidar to improve prediction accuracy. Alexandre Sauvage, Head of Leosphere, anticipates that “this approach is really appealing for most large airports and would increase capacity and safety”.
Supporting ATM demand and capacity balancing
Balancing demand and capacity is becoming a critical concern given the continued growth in air traffic, yet demand and capacity imbalances are difficult to predict, mainly due to the lack of accurate 4D trajectory information prior to daily operations. Future trajectory-based operations (TBO) will allow the coordination of 4D trajectory predictions and constraints across all operational stakeholders improving throughput, flight efficiency and punctuality. However, we are some years away from such an ideal position.
A promising application of ML to improve prediction of take-off times to enhance the accuracy of a ground-based prediction of an aircraft’s 4D trajectory (three spatial dimensions plus the time dimension) has been the subject of a study by EUROCONTROL in 2019.
At present, the most accurate estimated take-off time (ETOT) for each individual flight is provided by the network manager’s enhanced traffic flow management system (ETFMS).
The ETFMS messages are sent to Air Navigation Service Providers (ANSPs) for flights entering their airspace and to aircraft operators (AOs) by the Network Manager Operations Centre (NMOC) each time there is an event triggering a recalculation of an aircraft’s flight data.
In reality, aircraft do not always take off according to the ETOTs reported by the ETFMS due to several reasons including congestion, bad weather conditions at the departure airport and reactionary delay. The ML prototype developed by EUROCONTROL takes into account several of these factors in order to improve the take-off time prediction of individual flights before their Estimated Off-Block Time (EOBT).
Early successes
Two early successes include predicting passenger transfer connections and 4D trajectories.
London Heathrow Airport deployed a ML tool following a successful study, led by EUROCONTROL in early 2016 with the cooperation of University College London (UCL) and Darden Business school. The related decision support tool enhances key passenger transfer processes through data-driven predictions and the associated ML algorithm.
A second success story is in the EUROCONTROL Maastricht Upper Area Control Centre, where ML was used to predict 4D trajectories.
Project Manager Herbert Naessens said the 4D trajectory includes aircraft position, altitude and speed and already provides more accurate predictions. “We have an advanced prototype which we are considering for operational implementation.”
Results to date show a benefit of more than 47 per cent for horizontal accuracy improvement, while the average accuracy of the vertical predicted trajectory improves by more than 27 per cent – significant results from data-driven predictions.
What have we learned?
While there is plenty of justification for the optimism surrounding ML, things are not always straightforward. Current models are unable to identify all interesting patterns and interactions between the input features, in part because they are heavily dependent on available data, which often has accuracy issues that need to be resolved before use.
Nevertheless, the predicted take-off times produced by the model (trained with three years of historical flight and weather data) showed a reduction of the take-off time prediction error of approximately 30 per cent, compared to ETOTs reported by the current ETFMS. Ensuring a complete dataset and reducing the number of missing features could signifi cantly improve predictive accuracy beyond the current 30 per cent of improvement.
For the turnaround example, missing features correspond to those related to catering or boarding issues, aircraft maintenance and mechanical problems, crew schedules, passenger connections, airport runway configuration, and occupancy of parking stands, as well as other information specific to airlines and airports.
The challenge today is to build confidence with stakeholders and to share such data through acceptable confidentiality policies and technical infrastructure. The benefits to those stakeholders go beyond the challenges of data protection.
Another important learning factor is to be able to understand the proposed model. Understanding the rules behind the decisions of a model increases trust on its predictions, helps to figure out how the process being modelled works, and provides insights on how to improve the model’s performance.
However, the highest predictive accuracy is often achieved by complex models, such as deep artificial neural networks, where the most influencing features and the interactions between them can be difficult to understand.
Part of EUROCONTROL’s research is focusing on this challenge, ensuring a model can identify the most important features, the interactions between them and how they impact the final outcome.
These are just some of the issues to be considered when moving towards the regulatory approval of a data-driven ATC decision support tool.
How can we safely transition data analytics into ATM?
While real-time ML predictions already provide benefits for airport operations and ATC, this is primarily in non-safety critical contexts such as post-operations analysis or planning tasks. The future transition of data analytics to operational use in a safety critical environment, such as live runway operations, will come with challenges and risks.
ML-based predictions incorporate a level of uncertainty, so it is important that users are properly trained and safety nets are in place in case things go wrong. Relying on predictions means they may become a critical point of failure, leading to situations where a controller cannot efficiently operate anymore when a ML system fails.
In simulations, controllers expressed concerns about trust, workload issues and were apprehensive about the accuracy of the information provided to them. These are key issues to be addressed to ensure trust can be established before integrating ML into live operations.
On 23 May 2019, over 400 managers and experts from the European aviation community participated in the inaugural conference on aviation and AI, organised by EUROCONTROL in collaboration with Airbus. The conference highlighted that AI is already delivering promising results, but also that there is a need to coordinate aviation initiatives at large and to address fundamental issues that are impeding the development of AI.
EUROCONTROL proposed to set up a ‘European Aviation AI High Level Group’ with key representatives from all aviation sectors (airlines, airports, ANSPs, EU bodies, military and staff associations). The main objective of the European Aviation AI High Level Group is to develop concrete recommendations and a corresponding roadmap to strengthen AI in European aviation.
Future work
It is clear that ML systems have great potential in ATM, specifically in areas that involve decision making under uncertainty – for example, runway excursions or abnormal AROTs, queue management in terminals or taxiway optimisation. Research is also using ML to predict runway configuration changes to mitigate the impact on airport capacity, taxiing times, the occupation of parking stands and taxiways, as well as on the management of traffic in the airspace surrounding the airport, when a runway is changed.
There are significant opportunities linked to Air Traffic Flow Management applications, preparing for a day of operation by using historical data to provide a better understanding of expected scenarios linked to capacity, safety, runway and airspace configurations – resulting in timely decision making to adapt operations, implement mitigations and, potentially, improve safety.
But other non-safety areas – such as flight plan processing, environment, CNS monitoring, cyber-threats, just to mention a few – are areas where results can be quickly transitioned to implementation with significant benefit.
Is it safe?
Data analytics is a dynamic new thread in aviation and, despite some of its challenges, it promises to become an integral part of ATM operations in the future. As a result, a highly automated environment capable of supporting high intensity, complex operations will evolve, providing accurate decision-support tools to operators with the potential to transition aspects of ATM and ATC to greater automation.
However, we need to be vigilant, as unsupervised ML can lead to unexpected results.
A number of conferences have highlighted a list of technical and institutional prerequisites to deploying safety critical data analytics into the operational world. These include:
- Human acceptance and ethical issues
- Governance in terms of data provision agreements and equitable use
- Continuous accessibility
- Quality assurance of shared data
- Safety with regard to the applications that manipulate the data, such as ML and AI
- Validation/verification to a defined safety standard for the design case
- Certification, especially in a ‘live working context’
- Cyber-security – perhaps one of the most challenging aspects.
Typically, a ML algorithm is verified using historical data separate to that used to train the algorithm. However, the above-mentioned issues are pertinent depending on the final safety criticality of the defined use case. Where safety is a prime concern, the challenges listed above should be systematically and formally addressed in the safety argument prepared for the regulatory authority, prior to operational implementation.
Data analytics is driving a bright future in aviation, and stakeholders should embrace the opportunities, be confident, share data and ensure full benefits can be realised for all stakeholders.
Issue
Related topics
Related airports
London Heathrow Airport (LHR), Paris Charles de Gaulle Airport (CDG)